- Supervised learning (parametric/non-parametric algorithms, support vector machines, kernels, neural networks).
- Unsupervised learning (clustering, dimensionality reduction, recommender systems, deep learning).
- Best practices in machine learning (bias/variance theory; innovation process in machine learning and AI)
The course also draws from numerous case studies and applications: how to apply learning algorithms to building smart robots (perception, control), text understanding (web search, anti-spam), computer vision, medical informatics, audio, database mining, and other areas.
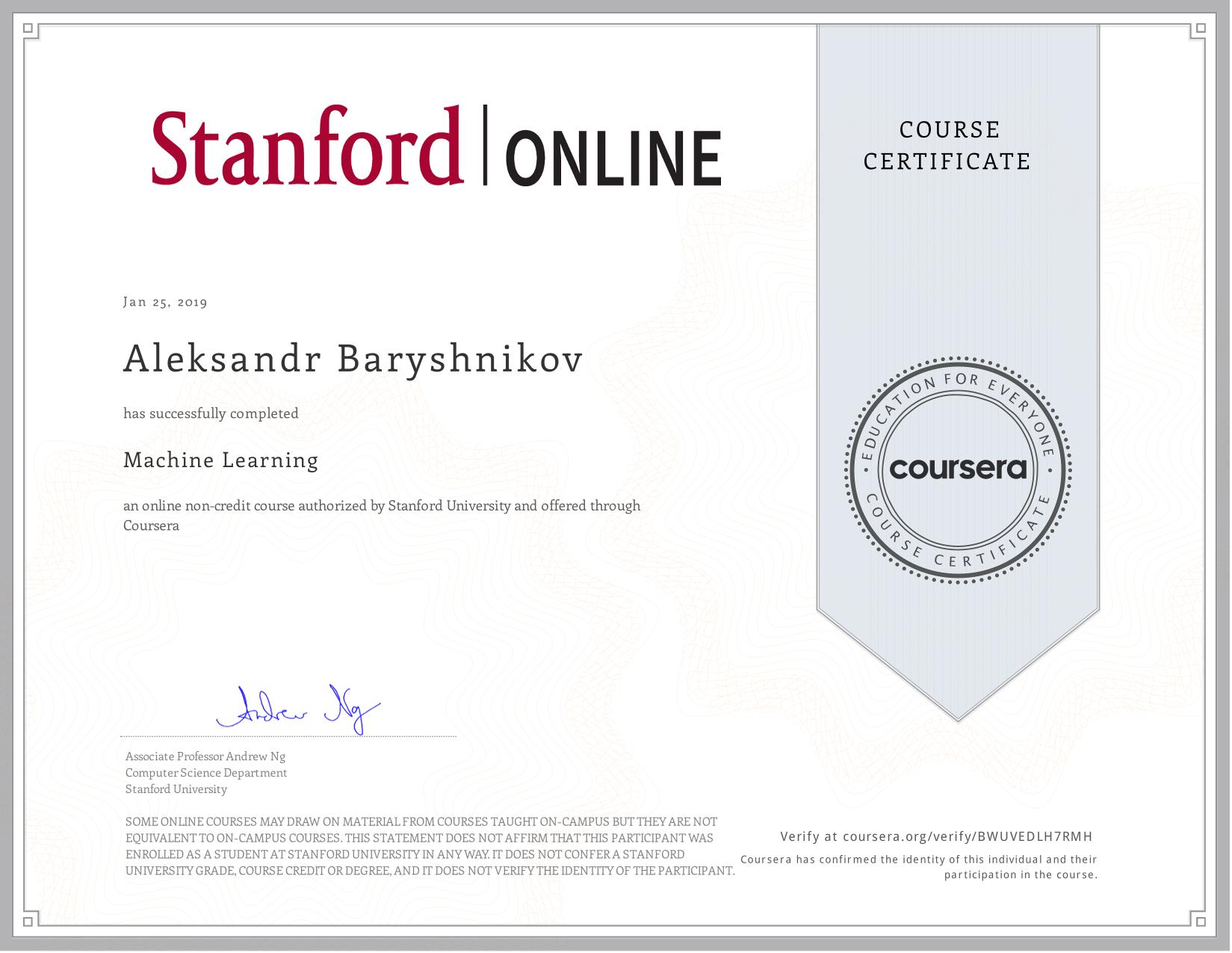
- Finished: 25 Jan 2019
- Certificate: BWUVEDLH7RMH